Recent years have witnessed remarkable progress towards computational fake news detection. To mitigate its negative impact, the researchers argue that it is critical to understand what user attributes potentially cause users to share fake news. The key to this causal-inference problem is to identify confounders -- variables that cause spurious associations between treatments (e.g., user attributes) and outcome (e.g., user susceptibility). In fake news dissemination, confounders can be characterized by fake news sharing behavior that inherently relates to user attributes and online activities. Learning such user behavior is typically subject to selection bias in users who are susceptible to share news on social media. Drawing on causal inference theories, researchers first propose a principled approach to alleviating selection bias in fake news dissemination. They then consider the learned unbiased fake news sharing behavior as the surrogate confounder that can fully capture the causal links between user attributes and user susceptibility. The researchers theoretically and empirically characterize the effectiveness of the proposed approach and find that it could be useful in protecting society from the perils of fake news.
Causal Understanding of Fake News Dissemination on Social Media
July 14, 2021
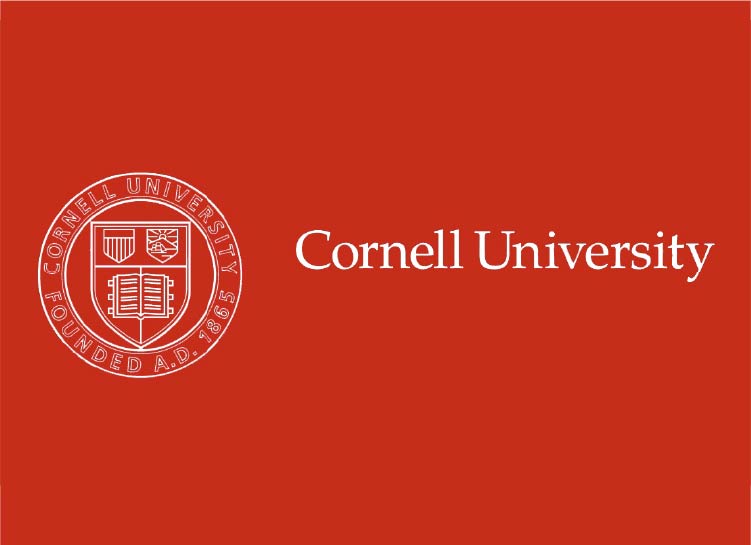