Researchers have raised concerns about the use of face recognition for, inter alia, police surveillance and job candidate screening (Deborah Raji et al., 2020). For example, HireVue’s automated recruiting technology uses candidate’s appearance and facial expression to judge their fitness for employment (Harwell, 2019). If a surveillance or hiring algorithm learns harmful human biases from annotated training data, it may systematically discriminate against individuals with certain facial features. We investigate whether industry-standard face recognition algorithms can learn to trust or mistrust faces based on human annotators’ perception of personality traits from faces. If off-theshelf machine learning face recognition models draw trait inferences about the faces they examine, then any application domain using face recognition to make judgments, from surveillance to hiring to self-driving cars, is at risk of propagating harmful prejudices. In human beings, quick trait inferences should not affect important, deliberate decisions (Willis & Todorov, 2006), but unconscious appearance biases that occur during rapid data annotation may embed and amplify appearance discrimination in machines. We show that the embeddings from FaceNet model can be used
Machines Learn Appearance Bias in Face Recognition
February 13, 2020
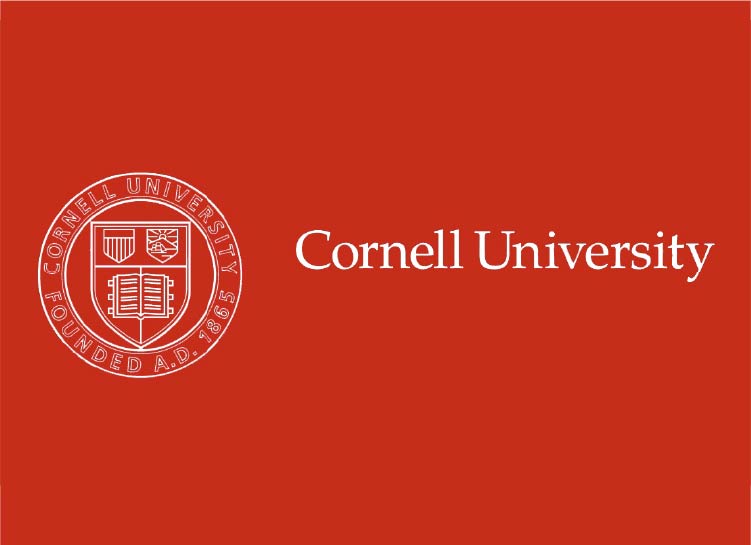